Up, down or out? Perspectives on artificial intelligence in health care today
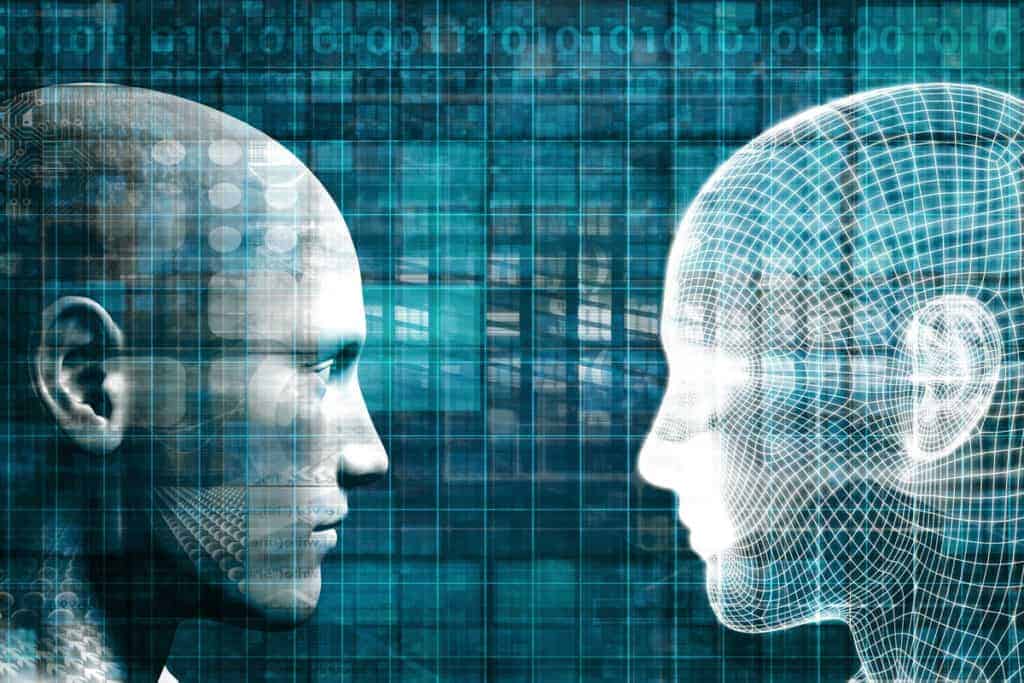
There are several high-value applications for artificial intelligence in health care. Challenges with underlying data sources and new ethical concerns have emerged as significant factors driving adoption.
Two-thirds of the attendees polled at a recent innovation summit by The Economist agreed on one thing: health care is the sector that will benefit most from artificial intelligence (AI) technologies.
In health care, which is in the midst of an industry transformation and a digitalization of key aspects of patient engagement and care management, the role of data, analytics and AI are central to the organizational mission. However, it is easy to get caught up in one aspect or another when extolling (or decrying) the role of AI, while ignoring the near-term potential as well as the limitations of the technology.
How can AI play a role in health care today? In the words of S. Somasegar, a venture capitalist, there are three ways in which AI can impact a business today. Upwards, meaning that AI can take on intelligent capabilities that enable a higher level of interaction with humans; downwards, implying an ability to reduce costs; and outwards, which is to take AI to the edges of our computing infrastructure.
Voice recognition and natural language processing (NLP) technologies help us move up in health care by enabling remote-monitoring and home health care through a “natural” interface with humans. In the health care enterprise, NLP technologies can “read” complex medical literature and provide doctors and clinicians with intelligent choices for diagnosis and treatment options.
With the emergence of cheap computing and storage infrastructure, AI technologies help manage vast arrays of servers and networking equipment, detecting and remediating the most common problems without human intervention. “Purpose-built” hardware with inbuilt AI capabilities are becoming the norm in high-volume and time-sensitive operations that require running machine-learning algorithms on large data sets and doing it at low costs.
The notion of edge computing, a paradigm that takes analytics and AI to the edges of a computing infrastructure, has lately become important in the context of the Internet of Things (IoT) and smart devices. In health care, the proliferation of intelligent devices, in and out of hospital settings, has created many new opportunities. Tom Bianculli, Chief Technology Officer of Zebra Technologies, a firm that provides mobile devices, scanners and RFID-enabled tags used in hospital environments, talks about “digital diaries” that can log every minute and every second of a device’s operation in the context of patient care. Using a network of tags and near-field communication equipment, Bianculli is now able to track a mobile device in a caregiver’s hands as she makes her way through a hospital floor, recording and analyzing everything from her precise location to her pace of walking to the direction in which she is headed with the device. Extending it to outpatient or even home health care, the deployment of intelligent devices that can analyze data at the “end point” and sending it back to a back-end system can save lives by reducing the time involved in alerting caregivers to medical emergencies.
To some, all of this may sound futuristic. However, it doesn’t have to be complex use cases and high risk situations involving patient lives that determine whether AI is suitable for a health care institution. The vast majority of AI use cases involve “low-hanging fruit” that automates aspects of operations that are routine and repetitive in nature. AI can release humans from mundane tasks and enable them to work on more exciting and value-added tasks. In some industries with an acute shortage of skilled human resources such as health care, this may even be a necessity for long-term sustainability.
The use of AI technologies comes with responsibilities as well. In the wake of recent disturbing news about a driverless car causing a fatal accident and the alleged misuse of Facebook profile data to influence the last presidential elections, there was a somber tone to the discussion at The Economist event. The gathering of AI technologists and industry leaders using AI to advance their business goals paused to reflect on how AI can be force for good and bad. Among the concerns: AI technologies by themselves may not reveal any inherent biases, but may unleash all manner of biases that reflect the biases of the humans who design the systems. There is a growing sense that AI should be used not just for the right predictions, but also to make predictions for the right reasons. While AI is coming on par with humans in aspects such as reading radiology images, the same neural network algorithms have potential for discriminatory profiling based on facial recognition and other decisions that have implications for society. The usefulness of AI models also depends on the data sets: as an example, selective representation of demographic profiles in a data set can give rise to biased conclusions on populations represented by that dataset.
The underpinning of success with AI lies in the underlying data. Fortune 500 companies are spending up to 50 percent or more of their IT budgets on information integration today, and no sector is more acutely aware of this than health care, with its complex environment of proprietary electronic health record (EHR) systems and emerging data sources. Unlike in other sectors such as consumer finance and retailing which are long used to multi-channel engagement with customers based on an omni-data capability that can aggregate and integrate data from a wide variety of sources, health care remains more siloed today than any other sector. The implications for AI adoption are clear: it will be slower than in other sectors.
Finally, having the data and the AI capability doesn’t ensure improved quality or reduced costs in health care. You need intervention models in place to do something with the data and have care plans for doing the preventive intervention, which can be challenging if the data is incomplete (as often the case with EHR data) or outdated (as with health insurance claims data). In an era of high-volume and high-velocity real-time data, these limitations will restrain the adoption of AI technologies.
As computing costs drop and AI technologies mature, health care and other industries will have to invest and catch up or get left behind in the great digital transformation under way. As someone said to me, there is a penalty for inaction. That penalty may be too big a cost to pay for most enterprises today.
Originally published on CIO.com